Department of Electrical Engineering and Automation
Department of Electrical Engineering and Automation
The Department of Electrical Engineering and Automation, in short EEA, is an ecosystem where scientists and engineers from different fields of microsystems, electrical engineering and automation work together to solve the most challenging scientific problems in the fields of energy and environment, as well as health and wellbeing.
We offer excellent education and top-quality research conditions.

Research
The Department of Electrical Engineering and Automation (EEA) focuses on three research areas: Power Systems and Energy Conversion, Control, Robotics and Autonomous Systems, and Health Technology.
The department is a major player in Aalto University’s Energy Efficiency Research programme AEF.
Also the Aalto ePowerHub is part of the EEA department.
Power Systems and Energy Conversion
We develop new methods, devices, and systems for substantially increasing the share of renewable energy and improving energy efficiency, focusing on making renewables, energy storage, and digital services the essence of power systems.
Power Electronics
Our research and innovation efforts on industrial and power electronics are taking place at three complementary levels: algorithms, methods, and applications.

Electromechanics
Advancing research in the interdisciplinary field of electromechanics at three different aspects: theoretical, numerical, and experimental.

Metrology Research Institute
Professor Erkki Ikonen
Electric Drives
Electric drives play an important role, e.g., in a large number of industrial applications, electric and hybrid vehicles, elevators, and robotics. Electric drives are systems where the electric motors or generators are controlled by power-electronic converters (such as a frequency converter).

Power Systems and High Voltage Engineering
The focus areas of research in power systems and high voltage engineering are distribution networks, their system solutions and reliability engineering, power system components´ load capacity and ageing, control and monitoring systems including computer applications, automation and communication solutions, end use analysis and power quality.

Power and Energy Systems
Power and Energy Systems

Illumination Engineering
Lighting research group’s fundamental goal is to advance the research and education of illumination engineering and electrical building services.

Smart Building Technologies and Services
The aim of the group is to advance research and teaching within smart buildings.

Renewable Energies for Power Systems
The mission of the Renewable Energies for Power Systems group is to pursue groundbreaking work in the generation, transmission, distribution, management, and consumption of electricity.

Control, Robotics and Autonomous Systems
In these research groups, we develop generic methodologies and practices focusing on distributed and networked control systems, field robotics, intelligent robotics, robotic instruments, industry automation systems, and automation software for distributed systems.
Nonlinear Systems and Control
The research interest of the Nonlinear Systems and Control group lies in the intersection of Control theory and Machine Learning: We aim to synergistically combine tools from nonlinear systems, stability theory, robust & optimal control along with machine learning and optimization techniques, to create systems with provable guarantees on safety and performance.

Cyber-physical Systems
The cyber-physical systems group develops novel machine learning and control methods for networked multi-agent systems.

Automatic Control
The research done by the automatic control group is based on a firm knowledge of system theory, control engineering, simulation methods, optimisation methods, numerical algorithms, and so forth.

Autonomous Systems
The group's research focuses on heavy duty semiautonomous machines and autonomous mobile robotics, mainly in agriculture and forestry.

Information Technologies in Industrial Automation
The group's research focuses on the engineering of software-intensive automation systems.

Intelligent Robotics
Intelligent Robotics group performs research in robotics, computer vision and machine learning.

Robotic Instruments
The robotic instruments group develops miniaturized robotics and fine objects manipulation technologies.

Robot Learning
The Aalto Robot Learning (RL) research group operates in the intersection of artificial intelligence and robotics. In particular, we focus on reinforcement learning, robotic manipulation, decision making under partial observability, imitation learning, and decision making in multi-agent systems.

Mobile Robotics
The Mobile Robotics research group is led by Assistant Professor Tomasz Kucner.

Distributed and Networked Control Systems
We are now witnessing an explosion in networked systems: everything is connected and massive amounts of devices are required to be coordinated.

Health Technology
We develop technologies for improved and predictive diagnostic tools and efficient therapeutic methods and offer new analytical techniques for basic medical research focusing on making medical solutions that enable personalised treatments, homecare and data driven clinical decisions.
Electronics Integration and Reliability
The research in the Electronics integration and reliability unit is strongly multidisciplinary by nature. Our knowhow is based on materials science and, especially, on the understanding of interfacial phenomena between materials in heterogeneous systems.

Bionic and Rehabilitation Engineering
Bionic and Rehabilitation Engineering (BaRE) research group investigates engineering techniques for human-machine interfacing in order to support, augment and rehabilitate human motor function. Through advancements in basic physiology, motor control, and biomechanics, we tailor novel biosensing and control approaches, as well as design methodologies in order to push the boundaries of current state-of-the-art bionic limbs, exoskeletons and rehabilitation robots.

Electromagnetics in Health Technology
The group develops computational methods for multi-physics modelling of the human body.

Microsystems Technology
Microsystems technology is a truly multidisciplinary research area. It is based on physical and analytical chemistry, biology, microelectronics, materials science, physics and biomedical technology.

Sensor Informatics and Medical Technology
Research of the group focuses on sensor informatics, adaptive signal processing, data fusion systems, and machine learning (including AI), especially for medical applications. Other applications include smartphone sensor fusion, robotics, positioning systems, target tracking, biomedical imaging, and many other indirectly measured time-varying systems.

Studies
The department offers a Master’s degree programme in the above focus areas and is heavily involved in the teaching and education in other cross-disciplinary programmes as well. Department also participates in the school-wide doctoral education as can be seen in our Doctoral dissertations.
Automation and Electrical Engineering, Master of Science (Technology)
The Master’s Programme in Automation and Electrical Engineering prepares its graduates to perform in the intersection between hardware and software, ranging from the fields of Electrical Engineering and Energy sectors to Biomedical Engineering, Control Engineering and Robotics. Drawing from extensive research and fundamental theories in mathematical and natural sciences, the programme focuses on the applicable side of technologies, including topics like Autonomous Systems, Health Technology, Smart Grids and Renewable Energy Solutions.

Become a doctoral researcher at the School of Electrical Engineering
Doctoral education will prepare you for the most demanding expert positions in your field in the academia, companies and public administration. Start here if you’re interested in doctoral studies!

Latest news

Join us for the first Aalto Open Science Award Ceremony
All Aaltonians are welcome – no registration required!
Robotics needs safe behavior patterns
Robotics and autonomous systems are developing rapidly. Algorithms that withstand disturbances and uncertainties in the system model and environment are critical for development.
Open Science and Research Summer Conference 2024 will be hosted by Aalto University
Open Science and Research Summer Conference 2024 will be held at Aalto University’s Otaniemi campus and in Zoom 3.–4.6.2024. Please register by May 3.
Congratulations to the 50 year old Metsähovi Radio Observatory
Exactly 50 years ago on April 11, 1974, the radio telescope in Kirkkonummi moved for the first time, and since then it has gathered data from outer space 24 hours a day, 365 days a year.Events

Public defence in Networking Technology, M.Sc. Dariush Salami
Spectrum Aware Human Centric Sensing using mmWave FMCW Radars (title of the thesis)
Wappu 2024
Aalto University's vappu, May Day
Public defence in Automation, Systems and Control Engineering, M.Sc. Oliver Struckmeier
Brain-inspired representation learning in robotics for efficient and robust sensory processing and learning.
Aalto Open Science Award Ceremony, May 7, 2024
Join us in celebrating the winners of the Aalto Open Science Award 2023!Head of department
Professors and Lecturers
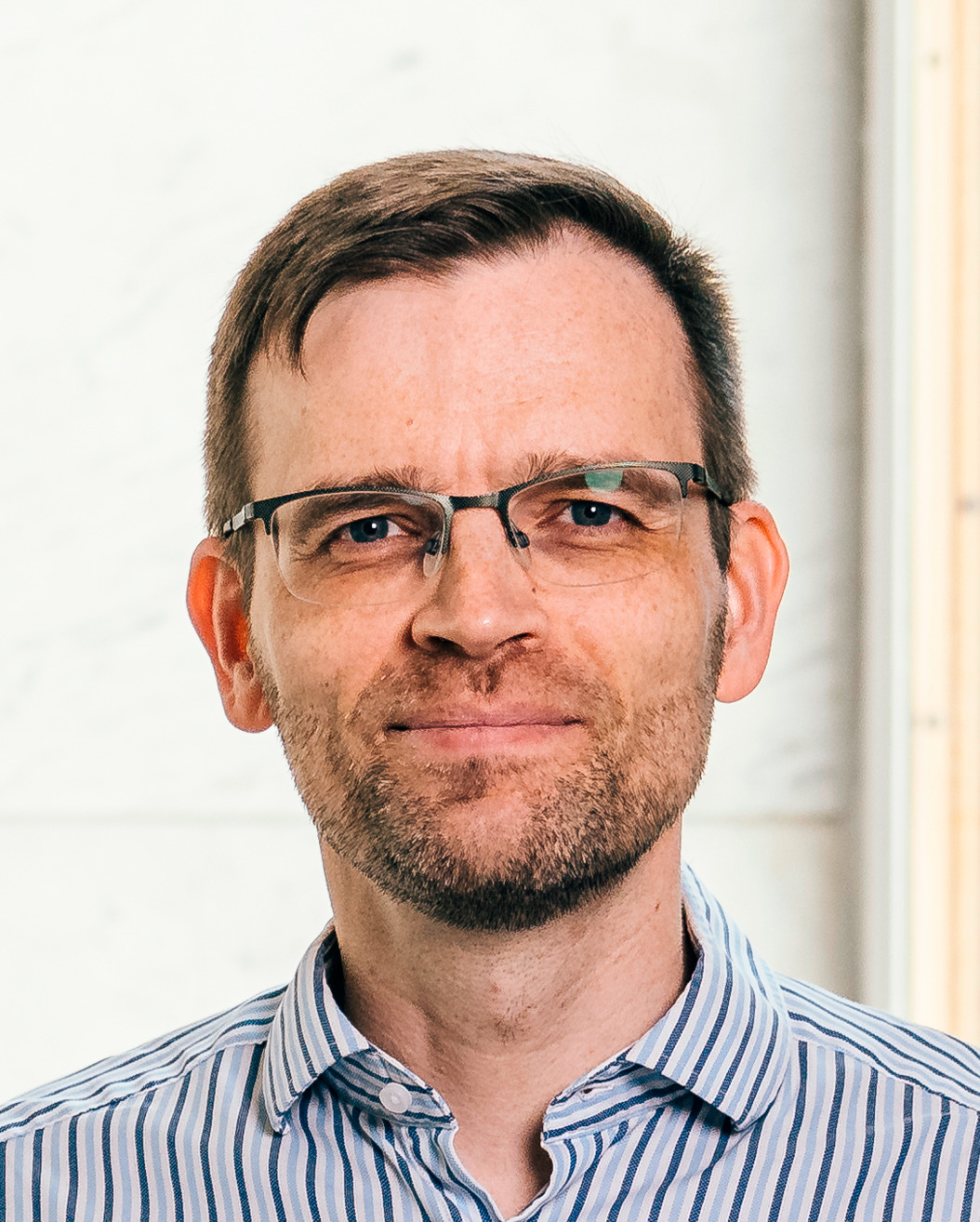
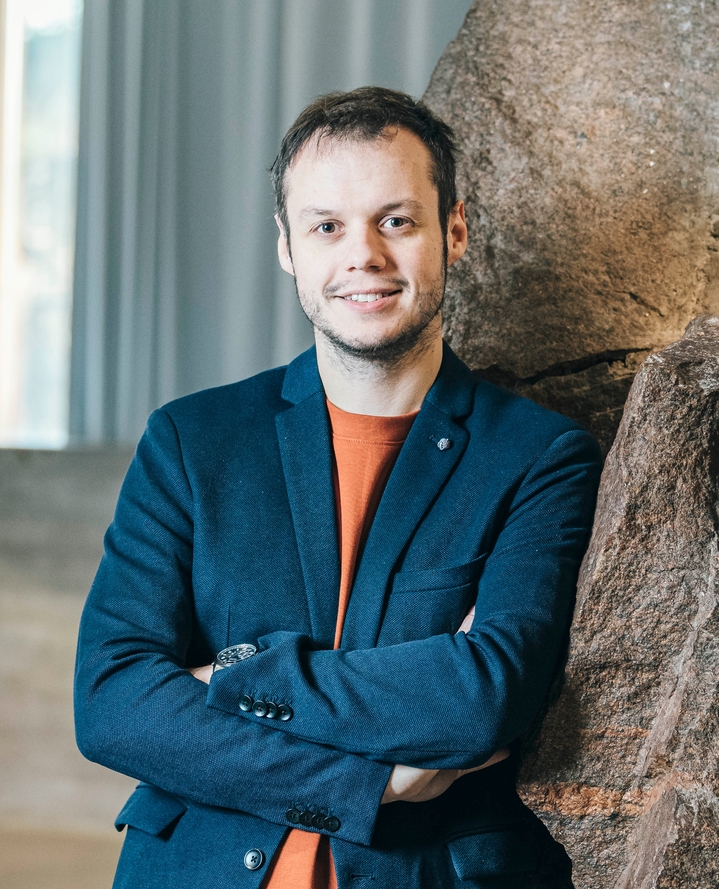
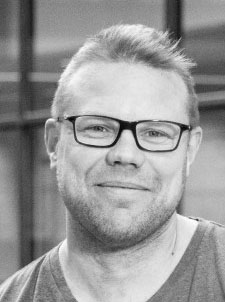
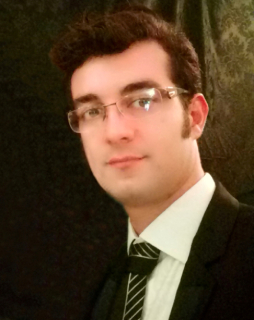
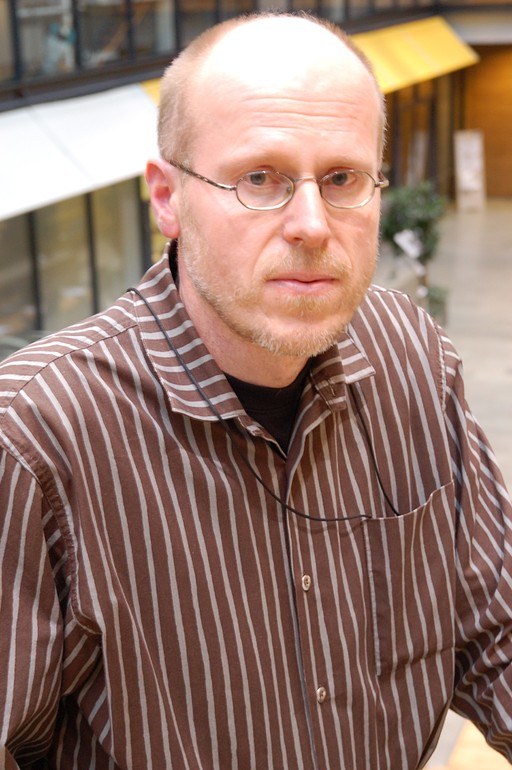
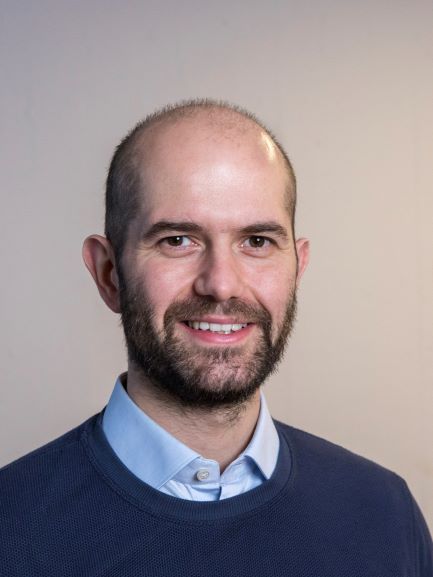
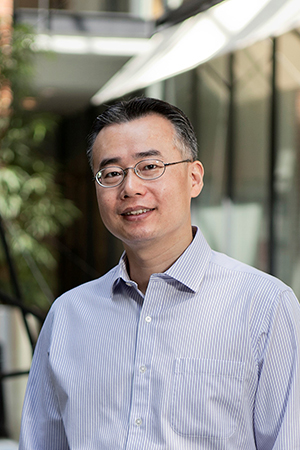
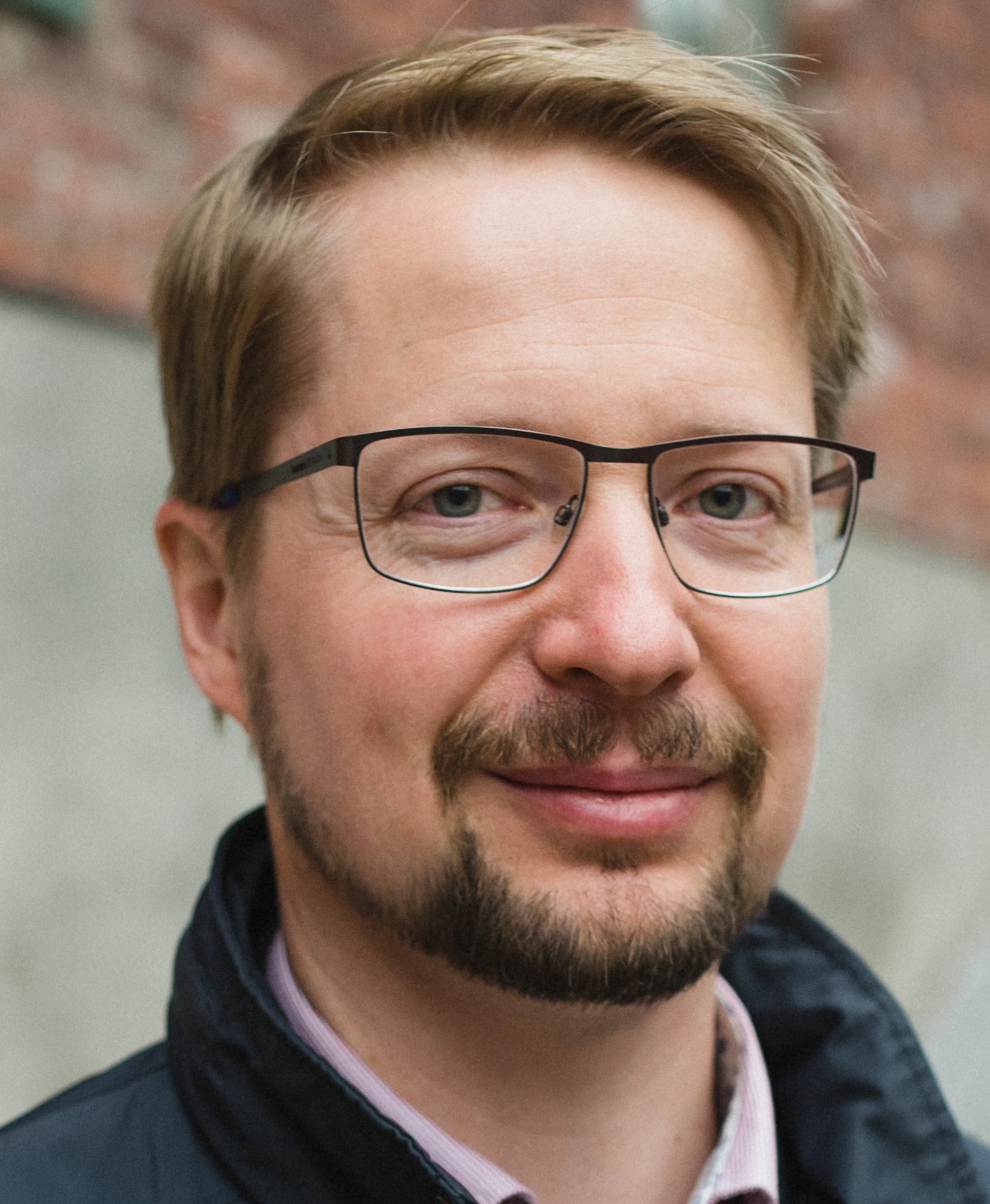
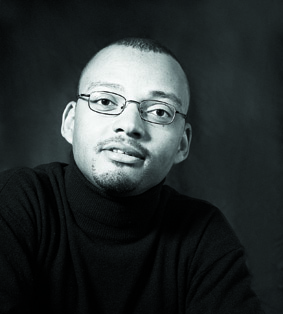
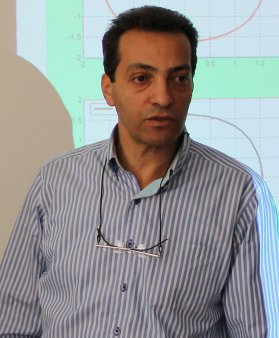
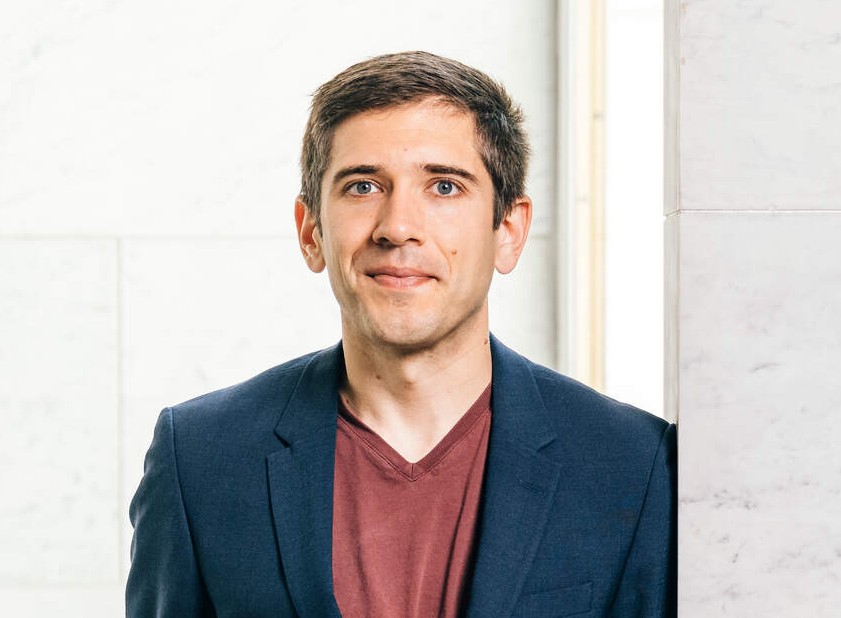
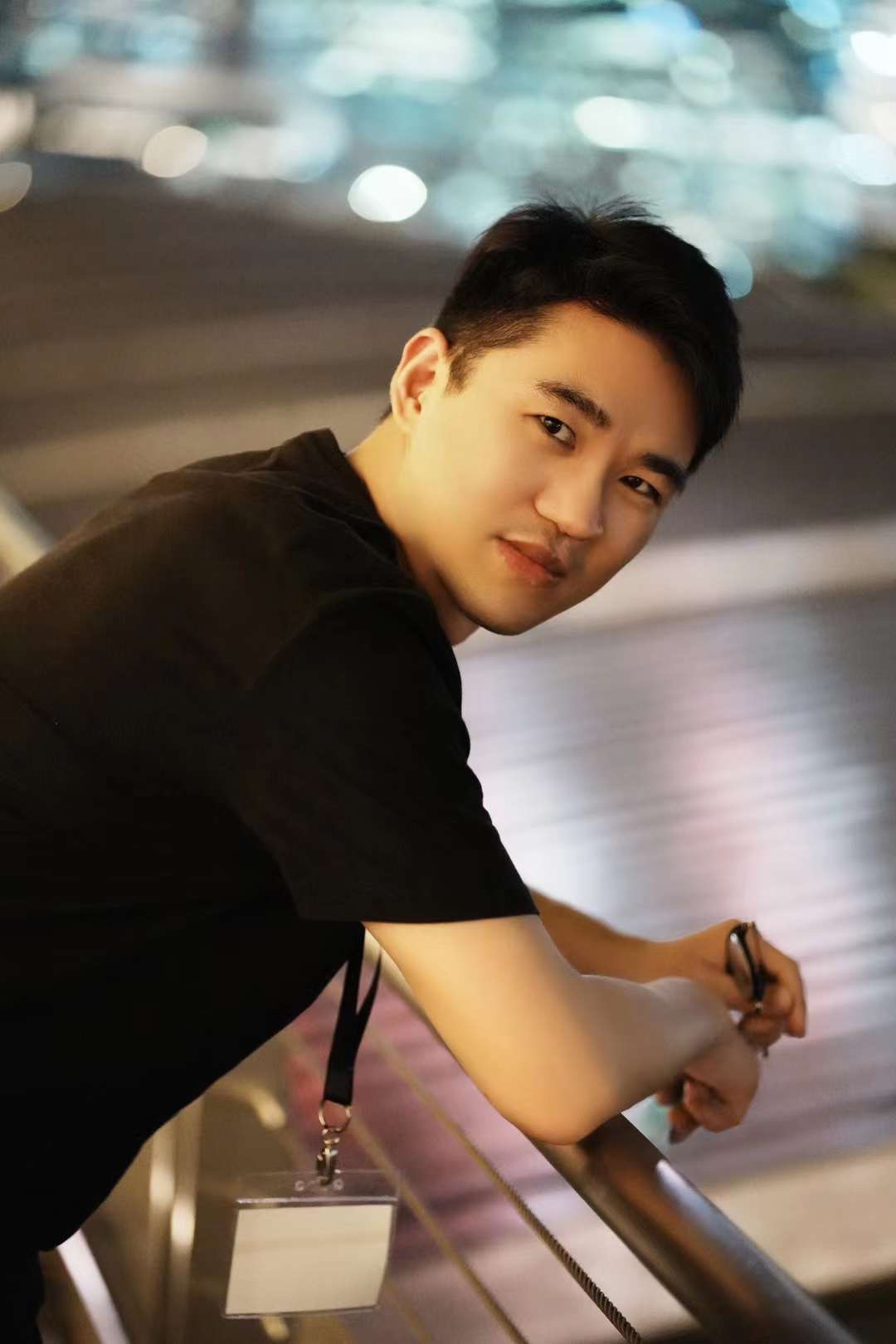
Where to find us
Maarintie 8
Maarintie 8

Health Technology House
Otakaari 3 & Rakentajanaukio 2

Micronova
Tietotie 3

Startup Hub A Grid
Otakaari 5
Open for members 24/7.

Aalto Factory of the Future
The Aalto Factory of the Future is an infrastructure to support innovation in future industrial automation, industry 4.0 & 5.0 and beyond. It allows test and trials of advanced technologies, such as future wireless communication (5G / Wifi6 and beyond) in industry.

Puumiehenkuja 5
Mechanical engineering 3, K3

- Published:
- Updated: